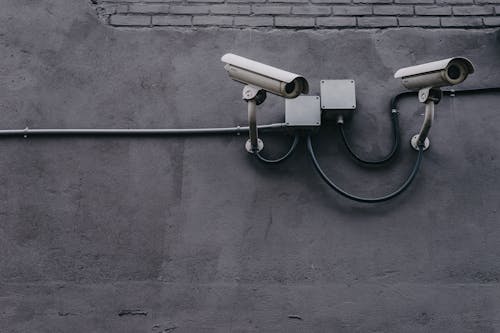
To what extent should we limit facial recognition technologies? In my February blog post titled, “Facial Recognition in 2020”, I asked what the “man in the street” thinks about facial recognition ending with, “Maybe in 2020 we’ll find out.” Well, I think we have. After the police killings of Ahmaud Arbery, Breaonna Taylor, and George Floyd, the public has made its voice heard in months of nation-wide marches for black lives and protests. Privacy, racial justice, and civil liberties organizations have at the same time called on government leaders to pass moratoriums on the use of facial recognition in policing. There is overwhelming evidence now of not only the racial bias that currently exists in facial recognition, but also growing awareness that even if the algorithms were completely unbiased, their use by law enforcement would still lead to unacceptable infractions on constitutional rights, especially in communities of color that are already oversurveilled and overpoliced. Respectable facial recognition companies concede that some sort of regulation is needed while privacy and racial justice advocates prefer outright moratoriums and bans, ranging from application only to law enforcement, to all government agencies, to use in all public spaces, to all uses period. How do we tackle this problem? To borrow from Microsoft President, Brad Smith, cleaver or scalpel?
First, the disagreement between advocates for “regulation around the edges” and those for complete bans in law enforcement or even more broadly stems from how companies and people will have different risk assessments. In turn, these perceptions of risk come from different experiences of those who make “the product” and think of it as something discrete to be engineered, packaged and sold and those who feel its effects as they move through the world. Of course tech workers are people too and also suffer from inequitable tech design, but we know that people of color are severely underrepresented in tech, so it’s likely that tech workers overall feel the burdens less. (Law enforcement and other organizations that purchase and deploy facial recognition of course also have their own risk assessments, but for the sake of brevity I’ll tackle that perspective in another post.)
Tech companies, like all companies, define risk typically from legal, regulatory, public relations, and of course business perspectives. They want to avoid producing technology that doesn’t work, doesn’t work well, or that people don’t enjoy using because it won’t sell. They want to avoid producing technology that can have adverse effects or cause harm so that they don’t get bad press or find themselves in court. These relatively narrow conceptions of risk inform the decision of whether or not to bring a given product to market. While some companies are starting to think more holistically about how their technologies will be (mis)used in the social environments in which they are deployed, this practice is still a bit foreign. On the other hand, people on whom the technology will be used assess risk through their lived experience of interacting with the technology. For example, Malika Devich-Cyril, founding director of MediaJustice writes in The Atlantic, “I’m a second-generation Black activist, and I’m tired of being spied on by the police.” Through her own experiences and that of her family history, Malika ties her social identity as a Black activist to her assessment of risk. And for this specific “user” the risk calculus weighs heavily on the side of an outright ban on facial recognition in law enforcement, not just because it’s biased against someone like her, but because the experience and feelings associated with constant police surveillance inform her understanding of how this new technology will be misused in an environment that already abuses power and authority.
So if corporations view the risk as relatively low (the product doesn’t work as well as it should) and citizens, especially those of color, view the risk as extremely high (routine infringement on basic constitutional rights all too often leading to arrest, imprisonment, or death but now aided by facial recognition), then of course the two sides will propose different solutions to mitigate that risk, each proportionate to their perceived level of risk. So the debate bifurcates into let’s keep using the technology because that’s the only way to improve its quality and let’s ban it immediately because in the meantime the risk to Black and Brown lives is too high, and even if the technology eventually improves to be less biased, the risk will continue to remain unacceptable.
Furthermore, we have to acknowledge, as sociologists frequently remind us, and what often derails the conversation around regulation, is that no technology (including facial recognition) is essentially good or bad, but rather it will be used for good or bad depending on the social, political, and cultural structures in which it is deployed, and of course what is deemed to be a good or bad use is culturally determined. The important point is that facial recognition is a socially crafted artefact that exerts power (in good or bad ways) over people, processes, and things leading to different decisions and outcomes than if it were absent. Many corporations tend to ignore this, instead designing, building, shipping, and measuring the return on investment of “the product”, as a discrete, unchanging unit that can be isolated from the world in which it is used. Citizens who interface with this “product” experience it not as a discrete piece of technology but rather as one that extends its effects into the depths of their daily lives and has material consequences. In the case of facial recognition in law enforcement, people of color often feel spied on, surveilled, stereotyped, profiled, and targeted. They experience the power of facial recognition as it sets into motion sequences of events that are all too familiar and predictable, ranging from incessant traffic stops, to illegal searches, to biased bail decisions, to longer sentencing… the cogs of the machine turn themselves, with considerable help from the new technology.
Scholars know this all too well from decades of research. Ten years after the publication of her book The New Jim Crow, Michelle Alexander warns that the use of AI algorithms in predictive policing is going to reproduce and compound racial injustice in the criminal legal system. Her prediction is based on her analysis of mass incarceration as a system designed to control Black and Brown bodies rather than prevent crime. If a technology is embedded into a social structure that is designed to create racial injustice and is very durable and immutable (despite concerted reform efforts), then the technology is likely to be deployed in the service of that design structure, despite its intended design. It alone is unlikely to carry enough weight to steer the tanker in the opposite direction. So while algorithms used in predicting crime are not necessarily themselves good or evil, they will have good or bad effects depending on the environment in which they’re put to work. Similarly, in Automating Inequality, Virginia Eubanks describes how welfare laws in Indiana designed to cut costs and move people off the welfare rolls were implemented via automated benefits eligibility screening. Proponents of the technology promised that it will remove human bias and increase efficiency. Instead, more people who did qualify were denied benefits, and particularly communities of color. The policy was designed by law to prioritize cost cutting instead of ensuring that everyone who needs benefits gets them and that’s exactly what the automated screening system ended up achieving, despite the tech advocates’ stated objectives.
More recently, we’ve seen similar examples in retail and healthcare. Rite Aid in recent days backed out of its use of real-time facial recognition technology in hundreds of its stores. Reuters revealed that the technology was predominantly deployed in poorer communities and communities of color. Rite Aid defended their plan by saying that it was “data-driven” and based on national crime data so facial recognition was deployed where “the crime” was happening. But to be truly data-driven you have to take into account that data themselves are not objective artifacts. Any Sociology 101 student could have consulted Rite Aid on the obvious biases underlying crime statistics in the US with respect to race, class, and gender. Any critical race scholar could point to decades of research on racial profiling in retail scenarios and how automated surveillance could reinforce (un)conscious bias. And any person of color could speak truth from their own lived experience. It is surprising that Rite Aid didn’t consider these structural issues in their risk assessment before rolling out this technology. Worse yet, maybe they did, but the decision makers abdicated their moral responsibility hiding behind “the data”. Rite Aid had to weigh the benefit to their bottom line against the harm of surveillance done to their customers, but to make an informed decision you have to put the data and your risk considerations in social context. And ultimately, the moral decision maker needs to be in the driver’s seat, not the data.
Finally, in a well-known case of racially biased risk scoring in healthcare, an algorithm developed by Optum was found to give higher risk scores to white patients than to Black patients, thus recommending disproportionately higher rates of white patients for receiving additional care and benefits over Blacks. Optum responded that the algorithm is not racially biased when predicting costs, which the research confirmed, and that is its intended and recommended use. But it is biased when misused to predict health risks or make health care decisions. Thinking about a single use case, it seems that Optum decided they had done a comprehensive risk assessment of “the product”, but they didn’t consider unintended uses. This is one of the unique difficulties with AI technologies; its effects can vary widely depending on how the technology is deployed and that is often largely beyond the manufacturer’s control. If Optum had considered the healthcare system as a whole and thought about the roles and incentives of the broader set of technology stakeholders (after all, doctors’ job is still to provide the best care possible not contain costs, right?) maybe they would have seen this coming and designed the system differently. Worse yet, maybe they did take unintended uses and their effects into account, but decided that their risk of liability for any harm done from using the algorithm “not as instructed” was low. Again, a technology will not be used according to its intended design (cost prediction) if the social system in which it is used is designed for other purposes (health prediction).
So where do we go from here? Let’s continue the conversation and pay more attention to the social structures within which facial recognition is deployed as that may help bridge the gap between different viewpoints on regulation and move us away from an unproductive is it good or bad type of discussion. As long as everyone’s voices are equally heard and everyone’s “risk profiles” are taken under consideration, then together we can arrive at the best solutions, ones that respect and protect all of us, and especially the most vulnerable. This is a tall order in an unequal society, but we have to try because ultimately whom we protect from risk and to what extent is a moral decision for which we are all responsible.